The Essential Role of Training Data for Self-Driving Cars
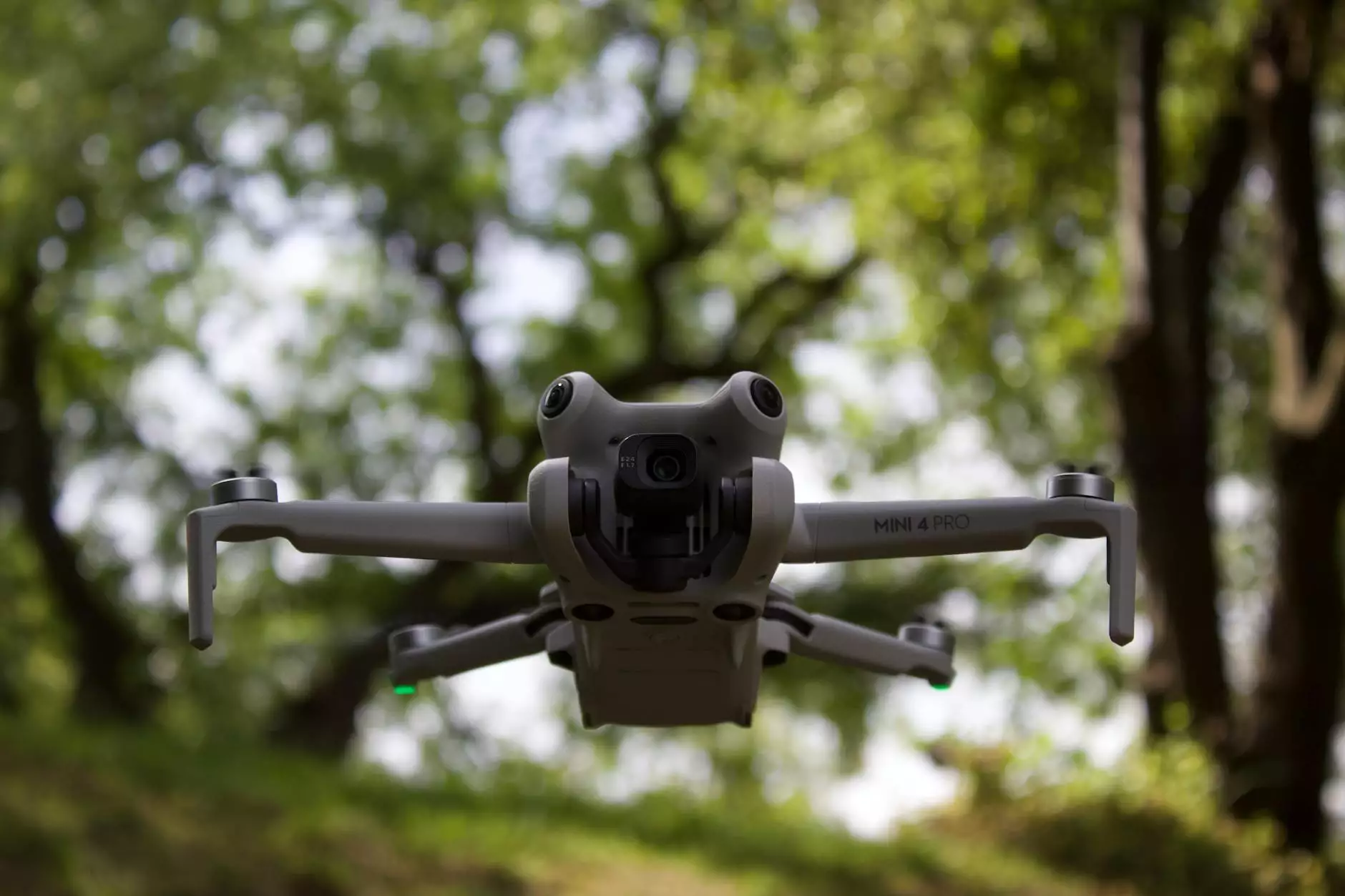
The evolution of self-driving technology has significantly transformed the automotive industry, introducing innovative solutions for safer and more efficient transportation. At the heart of this revolution lies an intricate process known as training data for self-driving cars. This article provides a comprehensive overview of what training data is, its importance, and how it shapes the future of autonomous vehicles.
What is Training Data for Self-Driving Cars?
Training data refers to the vast amounts of data collected to train machine learning models that drive self-driving cars. It encompasses various types of data, including:
- Sensor Data: Information collected from LIDAR, cameras, radar, and ultrasonic sensors that detect the car’s surroundings.
- GPS Data: Geographic positioning information essential for navigation.
- Vehicle Dynamics Data: Data related to the vehicle's motion and response to driving conditions.
- Road Condition Data: Information on the state of roads, weather conditions, and traffic patterns.
Why is Training Data Crucial for Autonomous Vehicles?
The effectiveness of self-driving cars heavily relies on the quality and diversity of training data. Here’s why it is crucial:
1. Ensures Accurate Perception
Self-driving cars must correctly interpret their surroundings to navigate safely. High-quality training data helps develop algorithms that allow the vehicle to recognize:
- Traffic signals
- Pedestrians
- Other vehicles
- Road signs and markings
2. Enhances Decision-Making Capabilities
Training data informs how a self-driving car makes decisions in complex situations, such as:
- Making turns at intersections
- Avoiding obstacles
- Responding to emergency vehicles
3. Facilitates Real-World Testing
To ensure safety and efficiency, self-driving cars undergo extensive testing in real-world scenarios. Training data helps simulate different driving conditions, including:
- Heavy traffic
- Adverse weather conditions
- Night-time driving
Sources of Training Data for Self-Driving Cars
Generating quality training data is a complex process. Major sources include:
1. On-Road Testing
Companies deploy fleets of vehicles equipped with sensors and cameras to collect data while driving on public roads. This method offers real-time insights into a variety of driving conditions.
2. Simulation Environments
Virtual simulations allow developers to create countless driving scenarios that would be difficult, dangerous, or impractical to test in real life. This can include:
- Extreme weather events
- Unusual road layouts
- Complex traffic situations
3. Public Datasets
Open-source datasets can also contribute to training data. Many organizations offer access to collections of data gathered from various environments and conditions, which can enhance the training models of self-driving cars.
Challenges in Collecting Quality Training Data
Despite the importance of training data, several challenges impede the collection of high-quality information:
1. Data Volume
The sheer volume of data required for effective training can be overwhelming. A single vehicle can generate terabytes of data per day, necessitating robust storage and processing solutions.
2. Diversity of Conditions
To ensure reliability, training data must encompass a wide variety of situations. This includes:
- Different geographic locations
- Varying traffic behaviors
- Seasonal weather changes
3. Data Labeling
The process of labeling data - identifying and categorizing information within the collected datasets - is time-consuming and requires accuracy to avoid potential errors in training.
The Future of Training Data in Autonomous Vehicles
The landscape of training data for self-driving cars is evolving. Advances in technology and AI are leading to:
1. Improved Machine Learning Algorithms
As machine learning techniques progress, vehicles can learn from smaller datasets and adapt more flexibly to new environments.
2. Enhanced Data Sharing
Collaborations between companies can lead to better data sharing practices, improving the overall quality of training data across the industry.
3. Increased Focus on Privacy and Ethics
As data collection expands, so do concerns about privacy. Future developments will likely prioritize ethical standards and transparency in data usage.
Conclusion
In conclusion, training data for self-driving cars is a vital ingredient in the recipe for developing safer, more effective autonomous vehicles. As we look to the future, addressing the challenges in data collection and enhancing the quality of training data will be paramount in realizing the full potential of self-driving technology. The integration of innovative machine learning techniques, collaborative efforts in data sharing, and a strong ethical framework will drive the industry towards unprecedented advancements in mobility.
For businesses in the automotive sector, understanding and implementing effective training data strategies will not only lead to better products but also foster consumer trust in autonomous technology. As these vehicles become ubiquitous, the role of training data will continue to be a cornerstone of this automotive revolution.
training data for self driving cars